Courses Offered by SPL Faculty
This research-oriented course explores advanced topics in management systems engineering, focusing on complex industrial, service, governmental, and healthcare systems. Students review state-of-the-art literature and develop research skills using a systems thinking paradigm to define and analyze efficiency performance measurement. Key topics include economic production theory, data-driven modeling techniques such as data envelopment analysis (DEA), statistical frontier analysis (SFA), and agent-based modeling. Through a translational research project, students apply theoretical frameworks to real-world socio-technical systems, examining the broader impacts and intellectual merit of efficiency performance. This course equips students with the analytical tools necessary for designing and improving system performance across multiple dimensions, including productivity, quality, and innovation.
Learning Objectives
- Develop an understanding of performance and efficiency measurement system design.
- Define system-level problems, identify their root causes, and model system structures influencing efficiency performance.
- Apply systems thinking and functional modeling to represent production systems, defining key inputs, outputs, and performance metrics.
- Analyze the impact of structural, organizational, and socio-economic variables on system efficiency.
- Utilize data envelopment analysis (DEA) and other quantitative frameworks to assess and improve system performance.
- Interpret model results, integrate decision support systems, and facilitate group modeling initiatives for organizational improvement.
This course provides a foundational understanding of socio-technical systems, integrating principles from systems theory, complexity science, safety science, macro-ergonomics, and policy design. Students explore the conceptualization, design, and management of socio-technical systems, with a focus on human-automation interactions in critical infrastructure, healthcare, and information systems. Through discussions, individual research, and team projects, students apply theoretical frameworks to real-world systems. Designed for graduate-level study, the course is ideal for students in industrial and systems engineering, as well as other engineering disciplines, preparing them to address challenges in automation-driven socio-technical environments.
Learning Objectives
- Identify and analyze key challenges in socio-technical systems.
- Critically evaluate different conceptual representations of socio-technical systems.
- Develop functional models defining inputs, outputs, outcomes, and contextual factors.
- Construct structural representations of socio-technical systems.
- Compare and assess various conceptualization frameworks, including DSRP, functional, structural, hierarchical, and complex adaptive system approaches.
- Apply theoretical concepts to real-world socio-technical systems through individual and team research projects.
This course presents principles that provide the foundation for the design of process-oriented efficiency measurement systems. Within this context, we introduce concepts that lead to the advancement of measurement science in management systems engineering. Furthermore, we present the link between the efficiency measurement literature and engineering systems. In this course, we focus on benchmarking (assessing) current efficiency levels and the planning (designing) for future efficiency improvement interventions. We base key concepts on an axiomatic framework that has its roots in microeconomic theory. Modeling approaches of efficiency and productivity measurement that we will cover include the following topics: index numbers, data envelopment analysis (DEA), statistical frontier analysis (SFA), and system dynamics modeling. We will demonstrate concepts by completing a research project.
Learning Objectives
- Learn the principles behind designing and applying performance measurement systems for process-oriented efficiency and productivity.
- Gain proficiency in various modeling methods, including data envelopment analysis (DEA), statistical frontier analysis (SFA), index numbers, and system dynamics.
- Assess current efficiency levels, identify peers, and define performance targets for improvement.
- Understand how efficiency and productivity relate to cost, revenue, profitability, and organizational policies.
- Develop skills to translate theoretical efficiency measurement principles into practical applications using empirical datasets.
- Explore how efficiency performance is influenced by structural, contextual, and behavioral factors within socio-technical systems.
- Conduct a research project utilizing efficiency measurement models and analytical tools like R to evaluate system performance.
Effective quality management is essential for the long-term success of technology-centric enterprises. This course explores the principles and strategies for developing, enhancing, and managing quality across all enterprise systems. Students learn to define quality, assess it using industry standards, compute quality measures, and design improvement strategies that align with broader performance metrics. Through case studies and a translational research project, the course provides practical insights into quality management systems, process optimization, and the impact of quality on cost, efficiency, and profitability. By the end, students are equipped to drive quality improvements in production, service, and managerial processes.
Learning Objectives
- Define quality management problems (knowledge and application outcomes).
- Specify quality dimensions and characteristics for technology-centric enterprises where inputs, outputs, outcomes, and contextual factors are defined for production and service processes based on quality management theory (comprehension outcome).
- Formulate models for quality management assessment (survey, qualitative, other) using quality standards with the translational research project (knowledge outcome).
- Compute quality management capability measures (analysis outcome).
- Design quality improvement plans with the translational research project (synthesis outcome).
- Assess the quality management performance of a production/service system with the translational research project (evaluation outcome).
- Relate quality performance to cost, efficiency, and profitability performance of technology-centric enterprises (analysis outcome).
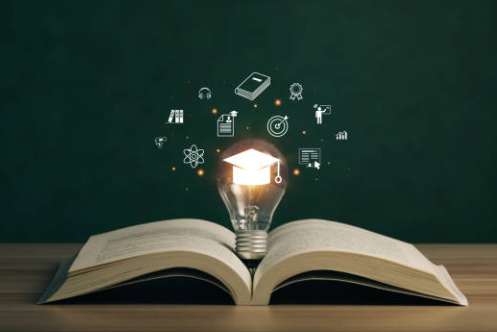
For more information about the courses, please contact Prof Triantis at triantis@vt.edu